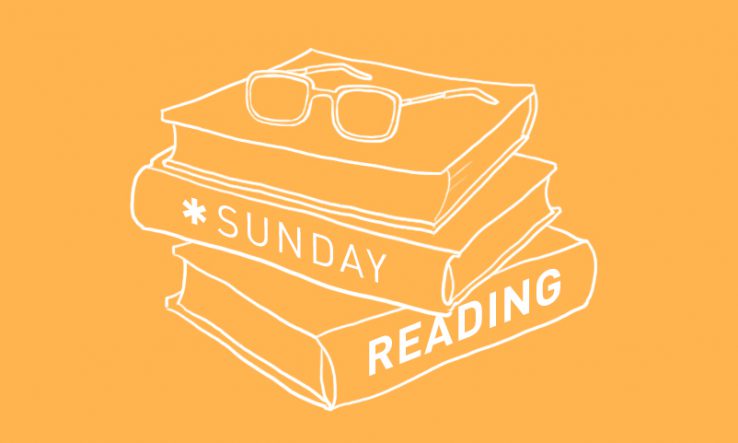
Vicki Stott argues that assessing higher education demands human, as well as artificial, intelligence
The rapid rise of generative artificial intelligence over the past 12 months has brought a wave of innovation, including in higher education. From rethinking the curriculum and evolving learning and teaching practices and assessment methods, to accelerating administrative tasks, the sector is embracing the opportunities offered by a technology that is likely to prove transformative.
Over the next few years, we can expect to see generative AI tools become more sophisticated and more deeply integrated into the standard software we all use. As a result, it is likely that the way students engage with, and learn in, higher education will change substantially by the turn of the next decade.
If AI transforms higher education delivery by 2030, how should external quality assurance develop?
Data analysis
First, AI offers the obvious potential to alleviate the burden on providers and quality agencies of collecting and analysing data and evidence. Integrated AI systems could allow a much smoother transfer of data from institutions to the quality agency or funder/regulator for the purposes of providers’ self-assessment, performance monitoring, or to identify areas to probe in more detail. With fast processing power and intelligent algorithms, AI could quickly and accurately identify trends and flag potential areas of concern.
With better data and evidence—and faster, less burdensome ways of analysing it— AI could support predictive modelling, helping to identify potential risks to the student learning experience or student outcomes before they materialise. This would combat the current issues with time-lagged outcome data and save time and resource in the long term by catching problems before they begin to have an impact.
The data capability that AI presents could also aid quality enhancement. Using data sets such as the National Student Survey, Teaching Excellence Framework submissions, course-level feedback, and cyclical review reports, AI could undertake extensive thematic analysis to identify common areas needing enhancement, around which an independent body such as the Quality Assurance Agency could then design a sector-wide enhancement programme.
Changed expectations
Much as digital and hybrid delivery has developed since the pandemic, high-quality AI-integrated teaching practices will emerge over the next few years. So too will common pitfalls that could threaten students’ learning outcomes. External quality assurance approaches play a role in identifying good and poor practice, and in supporting institutions to learn from both. This, in turn, develops understanding of what ‘high quality’ looks like, including in an AI-integrated higher education experience.
This principle also applies to standards. If, by 2030, human/AI hybrid writing is considered the norm, and assessment practices have evolved to acknowledge that, what effect might that have on the expectations we have of students around what they are capable of achieving? Quality approaches will need to verify that an institution’s provision is of the appropriate standard—and that standard might look and feel very different from standards today.
Trusted judgement
The value of any quality assurance exercise depends heavily on the level of stakeholder and public trust in its judgement. Should external quality assurance become AI-augmented, stakeholders will need transparency over how and why AI tools have been used throughout the process.
That said, we are likely to need to safeguard certain ‘uniquely human’ capabilities over the next decade to instil public trust in quality assurance outcomes. If technology-enhanced quality assurance approaches move faster than the sector and its stakeholders, it could undermine confidence.
Academic expertise and peer review are two core tenets of quality assurance approaches with human judgement at their heart. AI may be able to speed up, and make more robust, the quantitative analysis of the data that forms the basis of quality assurance information. But it is likely that more qualitative activities involved in review—such as considering the data against the context of a particular provider, understanding the way a provider operates on the ground, and engaging with staff and students—are better suited to human oversight.
Combining AI-augmented external quality assurance with defined areas of human oversight should provide richer and more robust assessments of quality that help providers know their areas for development and how to improve.
There is no doubt that external quality assurance will have to adapt alongside learning and teaching practices as AI transforms higher education over the next decade. But it will also be important to bear in mind the extent of public trust in AI-augmented aspects of quality assurance, so as not to undermine confidence in assessment judgements. If used well, AI could enable a robust and richer data landscape through which to understand quality and outcomes, thereby delivering high-quality learning experiences for students. To achieve that, balancing AI with human expertise will be crucial.
Vicki Stott is CEO of the Quality Assurance Agency