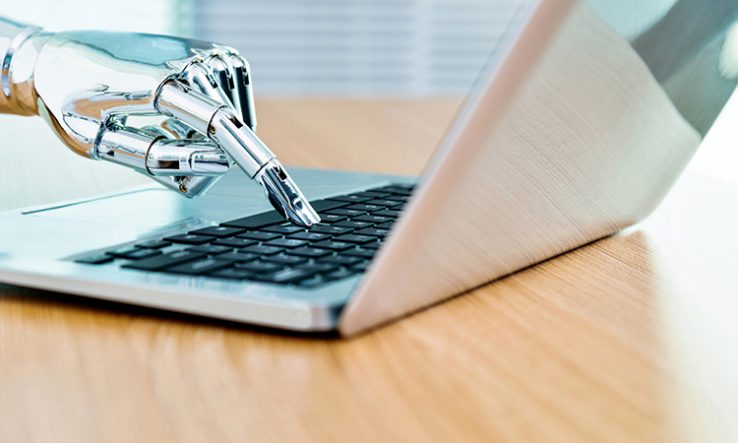
Age of algorithms challenges traditional notions of good research, say Wytske Hepkema and her colleagues
Citation recommendation tools are at the frontier of technology to automate the process of writing an academic article. These applications process textual input during writing—ranging from a single sentence to entire manuscripts—and recommend a fitting citation based on a learning algorithm. The ultimate aim is to automate the process of compiling a reference list, suggesting citations based on an author’s text.
This may seem like a great innovation that will save researchers a lot of time. We argue, however, that such tools encourage questionable citing practices and should thus be developed and implemented with caution.
Citation recommendation tools are still in their infancy. They have mainly originated in computer science, and require a certain amount of technical literacy and know-how to use. Most current attempts, such as Specter, created at the Allen Institute for AI in Seattle, are at the stage of models of how such tools should work. Some are supported by pilot implementations on limited datasets, but they generally lack user-friendly interfaces that support wider uptake.
But experiences with similar tools to automate the writing or reviewing of academic texts, such as plagiarism-detection software or programs to support statistical analyses, suggest that these tools might rapidly become user-friendly, commercial products. They are also likely to become part of the offering of large companies.
There are already many commercial tools that help researchers order their references, such as Mendeley from Elsevier, Clarivate’s EndNote, and Citavi from QSR. Some of these are increasingly linked to other services, becoming part of large portfolios of tools automating several elements of the research process, such as sharing references or supporting systematic literature reviews. (Research Europe is an editorially independent part of Ex Libris, which is owned by Clarivate.)
Before citation recommendation tools become widely available, and before the algorithms driving the recommendations become trade secrets, and thus black boxes, there needs to be a debate.
Skewed rewards
These tools run the risk of encouraging questionable citation practices, further undermining the purpose and value of citations in individual papers, potentially reducing the quality and reproducibility of research and—given citations’ role as a currency in the scientific recognition system—skewing rewards down the line.
One problem is simply that such tools allow authors to compile a plausible-looking bibliography while hardly reading the work they are citing. This risks exacerbating already flawed citing practices in science, and putting unwarranted responsibility on editors, reviewers and readers to vet reference lists.
For an editor, reviewer or reader to check all of a paper’s references requires a Herculean effort. The fact that many papers continue to be cited uncritically after retraction shows that such checking is often lacking.
But the problems go beyond sloppiness. Citation recommendation tools in their current setup aim to deliver mostly confirmatory rather than critical references. This is particularly problematic for some types of research, such as hypothesis-testing studies.
Culture of convenience
Ideally, researchers should do their reading before their research; a citation should show that a previous contribution has been digested and is being built on. But the design of these tools, as support for writing after the research is complete, encourages a culture of convenience rather than diligent, patient science.
As well as giving confirmatory references, citation recom-mendation tools are also inherently conservative. Learning from the past and mimicking current citation practices, they serve up what is already popular, making it more so.
This risks exacerbating existing biases in academic literature, such as the tendency for positive findings to attract undue attention, and science’s reward system. Since citation counts are often used in evaluation processes, including hiring and promotion, the tools risk increasing the existing bias in favour of popular articles and those from favourable sources, such as prominent institutions.
These biases are already baked into widely used academic search engines. But by taking automation closer to the heart of writing, citation recommendation tools run the risk of creating even stronger biases.
More generally, the design and use of these tools should be part of a broader conversation about automation in research. What is the line between labour-saving and cheating? What are scientists responsible for in their work? And what does it mean to be a scientist and do good science in an age of automation?
Wytske Hepkema, Willem Halffman and Freek Oude Maatman are at Radboud University in Nijmegen, the Netherlands. Serge Horbach is at Aarhus University, Denmark
This article also appeared in Research Europe